Welcome to xView3!
A competition to detect dark vessels using computer vision and global SAR satellite imagery
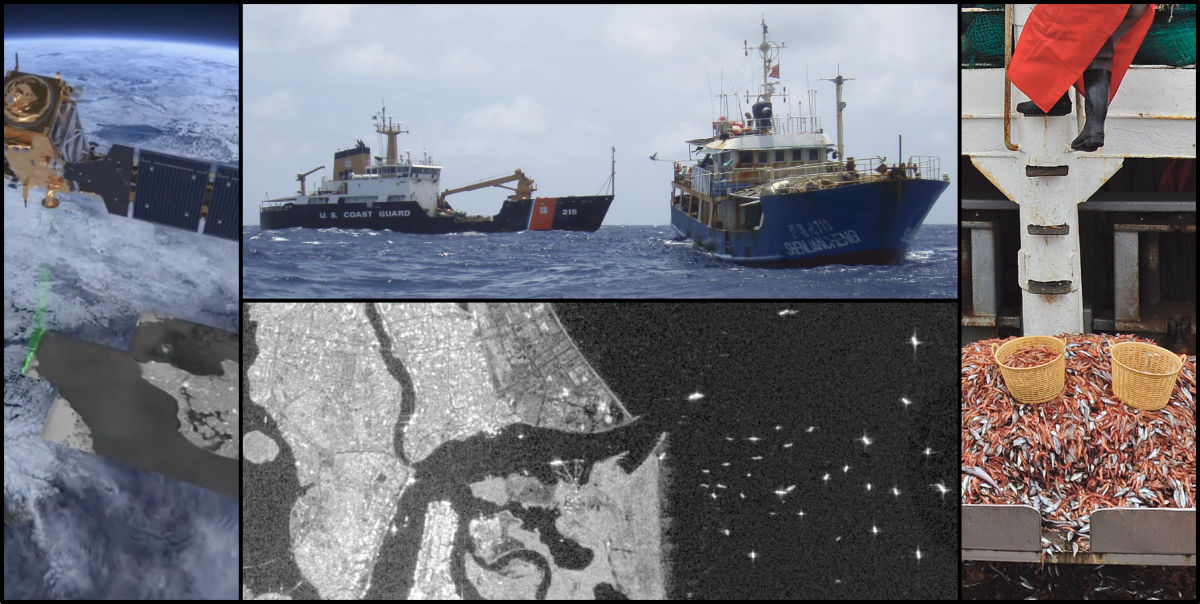
Image credit: U.S. Coast Guard; used with permission. ESA; image contains modified Copernicus Sentinel data (2020). ATG medialab; CC BY-SA IGO 3.0. Composites © Cambrio; rights reserved.
Winners
The winners have been announced by DIU and Global Fishing Watch. Congratulations!
The problem
Illegal, unreported, and unregulated (IUU) fishing is a major threat to human food supply, marine ecosystem health, and geopolitical stability. IUU fishing is widespread, threatening the sustainability of global fisheries in national waters and on the high seas. Developing countries are most at risk from IUU fishing, with estimated actual catches in West Africa, for example, being 40 percent higher than reported catches. Worldwide, one in five wild-caught fish is likely to be illegal or unreported; the econonomic value of these fish never reaches the communities that are the rightful beneficiaries. Annual global losses due to this illegal activity are valued at $10 billion to $23.5 billion USD.
The worst examples of IUU fishing are often connected to trans-national crimes, including human rights abuses, bonded labor, tax evasion, piracy, and drug, arms and human trafficking. IUU fishing also exacerbates the effects of climate change on ocean resources.
The trans-national nature of IUU fishing demands the ability to readily share and receive data among nations. More cost-effective tools for rapid detection of suspicious and illicit fishing activity will strengthen enforcement and control efforts, mitigating the damages caused by IUU fishing.
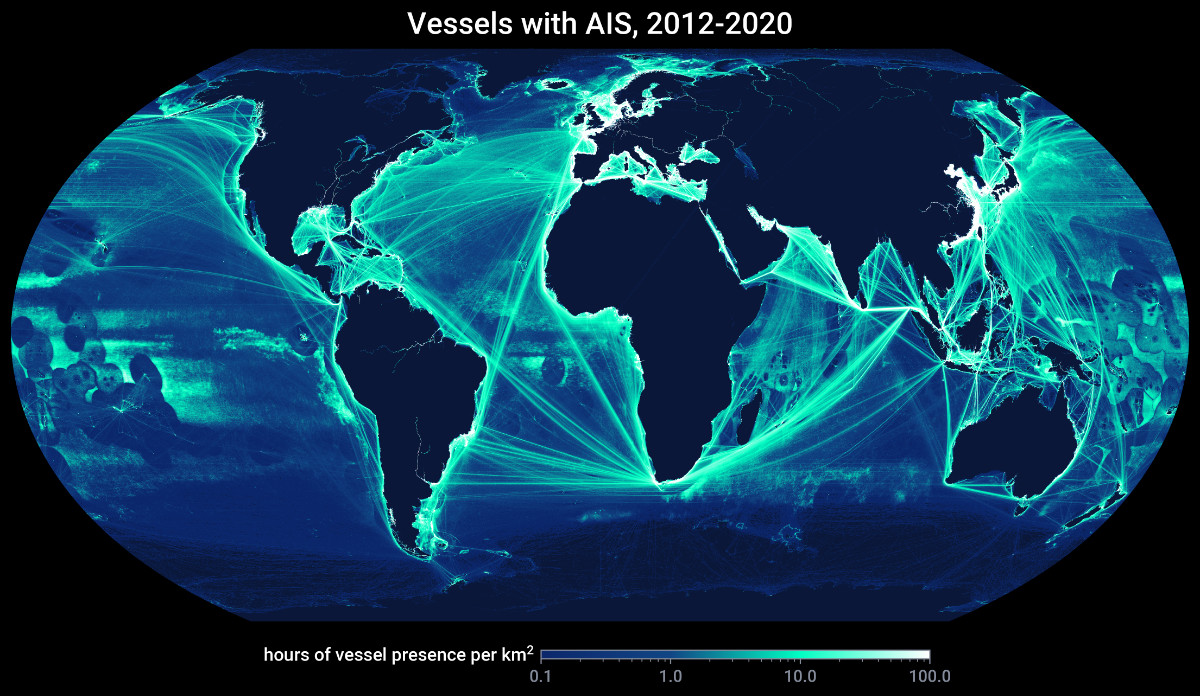
Image credit: © Global Fishing Watch, rights reserved.
Why is it hard?
For IUU fishing activity to be addressed and reduced, it must first be detected. While many tools have been developed to help detect and monitor IUU activity at sea, the current options all have limitations:
- Approaches which rely on cooperation from the vessel, such as radio transponder signals like the automatic identification system (AIS) and vessel monitoring system (VMS), are susceptible to manipulation and tampering by those who wish to conceal their activity.
- Passive satellite sensors, such as electro-optical (EO) imagery, which acquire the reflected electromagnetic waves of sunlight and/or the infrared radiation emitted by objects on the ground, are confounded by cloud cover, haze, weather events, and seasonal darkness at high latitudes.
Synthetic aperture radar (SAR) is one of the power tools of remote sensing, and an increasingly valuable complement to other vessel detection systems. Active satellite sensors, such as SAR, transmit radar waves to the Earth and measure the backscatter and traveling time of the signals that are reflected back from objects on the ground. Vessels at sea provide a strong reflection signal, giving SAR robust detection capability that is unaffected by clouds or weather.
SAR has proven to be the most consistent option for detecting vessel presence, as it does not require cooperation or cloud-free skies. However, analysts have traditionally struggled to glean useful information from SAR imagery for identification and classification of vessels for a variety of reasons, including: image resolution, sensor incidence angle, vessel build material, radar cross section, wind/wave conditions, and the lack of ground-truth data (such as AIS) to corroborate analysis results.
Moving from simple vessel presence and length estimates to reliable detection of vessels engaged in IUU fishing further complicates the challenge. Vessels must be categorized by type and activity (fishing vs. not fishing) and evaluated for the likelihood that any fishing activity is illegal, unreported or unregulated.
Our opportunity to solve the problem
Although SAR sensors have been providing satellite imagery for decades, the technical and economic barriers to improving analytical capabilities have proven to be substantial. There are no global, high-quality, consistently processed SAR datasets with validated ground truth detections available for academic, industrial, and open source communities to tackle the problem of detecting and classifying the activity of vessels at sea.
For xView3, we created a free and open large-scale dataset for maritime detection, and the computing capability required to generate, evaluate and operationalize computationally intensive AI/ML solutions at global scale. The data are consistently processed to include aligned views and relevant context above and below the ocean surface, with ground truth detections derived by combining AIS tracks, existing automated SAR analysis, and human visual detections. We believe it to be the biggest and best dataset of its kind, and are eager to see it in action throughout the community!
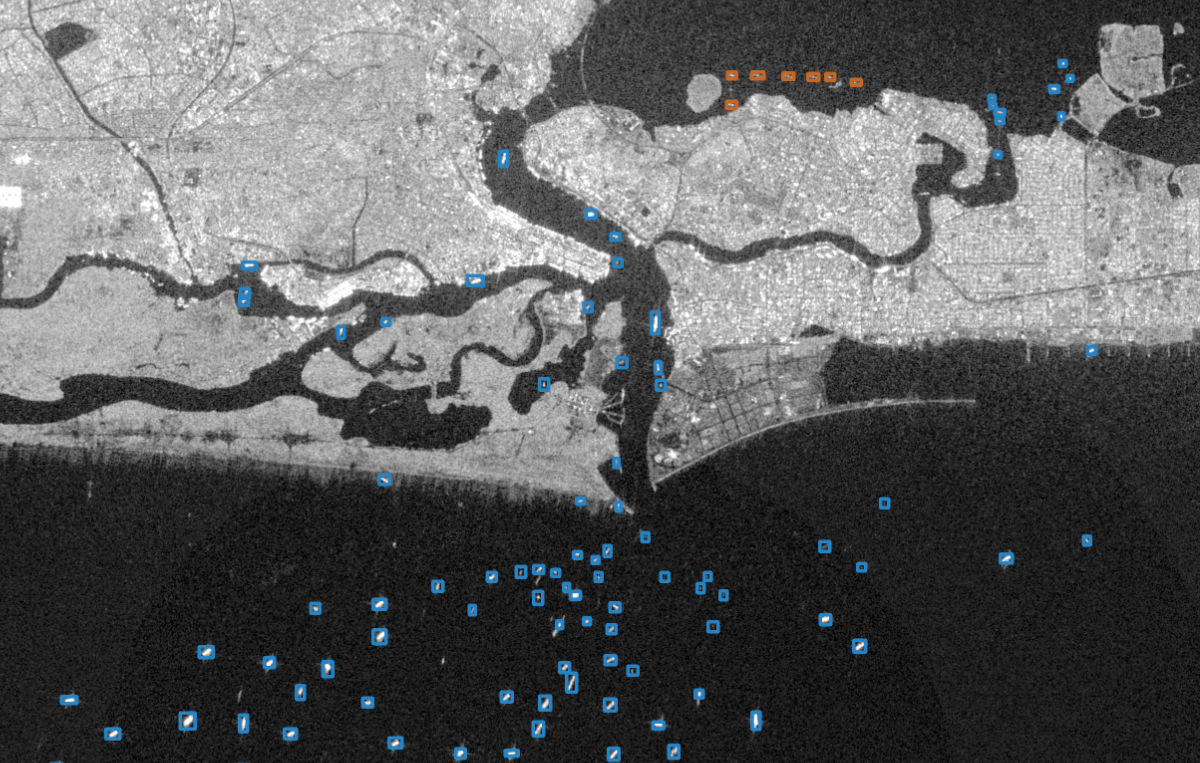
Image credit: © Cambrio LLC; rights reserved; image contains modified Copernicus Sentinel data (2020).
Why have previous approaches failed?
Previous attempts to use automated algorithms (e.g., artificial neural neworks) to detect IUU fishing activity from overhead imagery have been limited by a lack of both available remote sensing data and data labeling capacity, which requires subject matter expertise, large amounts of raw imagery, and the technical capability to both process the imagery and match historical AIS signals to views.
Archival imagery from existing commercial SAR sensors in orbit is difficult to access in sufficient volume to train a sophisticated algorithm. The data are prohibitively expensive, subject to end user license constraints, sporadically available in a given area of interest, and generally delivered in a context that requires human analyst review. This limits scalability and prevents a transparent way to evaluate the accuracy of the underlying automated solution.
The xView3 challenge leverages labeled training data derived from the combination of Global Fishing Watch's historical detection model for maritime objects, the output of its unique AIS analytics, and human visual detections. SAR imagery is derived from the European Space Agency’s Sentinel-1 satellites, a non-commercial option with an extensive global archive. This competition aims to stimulate the development of applied research in detection algorithms and their application to commercial SAR imagery, thereby expanding detection utility to greater spatial resolution and areas of interest.
What is xView?
xView is a series of international computer vision competitions run by the Defense Innovation Unit, to advance, benchmark, and procure state-of-the-art computational solutions in domains relevant to national security. We have partnered with Department of Defense organizations, federal, state, and local first responders, and non-governmental organizations to create and release big, high-quality, open datasets aligned to specific prediction tasks that are relevant to national security and the world at large.
The most recent xView competition, xView2, sourced computer vision solutions to automate building damage assessment, using satellite imagery from before and after natural disasters:
- Over 2000 submissions resulted in three winning solutions, with 80% damage detection success rate
- The top solution was 266% better than the government baseline algorithm
- Winning solutions were deployed to assist with a variety of natural disasters around the world in 2020 and beyond, from the Australian bushfires, to seasonal wildfires in California, to hurricanes and floods in the Southeastern USA.
What is the anticipated impact?
xView3 builds on the success of the preceding xView challenges, to:
- Advance the state of the art in automated detection of fishing activity from SAR imagery;
- Increase the number of machine learning researchers working on maritime SAR imagery, by eliminating a key blocker---availability of a high-quality labeled dataset suitable for algorithm development;
- Provide objective benchmarks by scoring submissions against hold-out data, thereby characterizing the level of performance for state-of-the-art solutions on this prediction task;
- Procure winning algorithms as open source solutions, or where appropriate, utilize IAW 10 U.S.C. § 2374 Prize Challenge Authority
Who is Leading xView3?
The competition is organized and run by:
- Defense Innovation Unit (DIU), a United States Department of Defense organization accelerating the adoption of commercial technology to advance national security.
- Global Fishing Watch (GFW), an international nonprofit organization dedicated to advancing ocean governance through increased transparency of human activity at sea.
Additional funding and support is contributed by:
- U.S. Coast Guard (USCG), one of the primary U.S. organizations responsible for combating IUU fishing, often in collaboration with international partners.
- National Maritime Intelligence-Integration Office (NMIO), U.S. agency that integrates maritime intelligence, improves information sharing, and fosters domain awareness to protect the United States, its allies, and partners against threats to, from, and in the global maritime domain.
- National Oceanic and Atmospheric Administration (NOAA), a scientific agency in the US Department of Commerce focused on the conditions of the oceans, major waterways and the atmosphere.
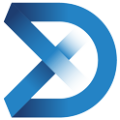
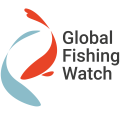
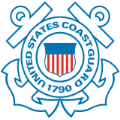
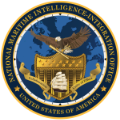
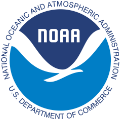